Check out the latest article from Forbes Tech Council featuring our founder
Balaji Ramadoss. In a recent Harvard Business Review article entitled "Why Data Science Teams Need Generalists, Not Specialists," Eric Colson defines the limitations of traditional “division of labor” structure as it pertains to data science and compares it with a “learn as you go” structure facilitated by the generalist. The division-of-labor model works well, he notes, when the requirements “fully describe all aspects of the product and its behavior.” But when “knowledge” is the requirement, the specialization construct found in the division-of-labor models only hinders. As a result, Mr. Colson describes the need to balance learning vs. efficiency gains by hiring full-stack data scientists -- generalists who are responsible for everything from conception to implementation.
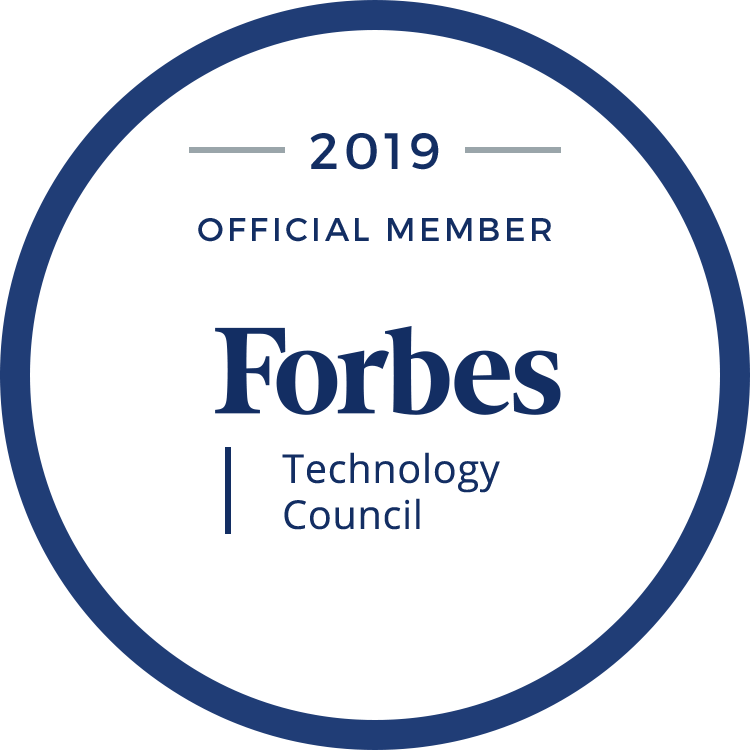
Today, information technology and data platforms perpetuate the barriers for operationalizing with architectures that mimic the silos based on the function-based division of labor. Contemporary data platforms are designed around these function-based divisions that have not evolved much since the 1990s. As an example, storage and normalization specialists are still the bottlenecks for the as-a-service platforms of today, mimicking the traditional silos in specialization.
Bloated investments in data architectures such as multiple warehouses, on-premise data marts, cloud-based data lakes and disparate business intelligence tools tend to result in data remaining mired in their operational silos. This trend is observed across all industries and, in many cases, business lines within industry verticals tend to have different solutions perpetuating the need for specialists to care and feed data and technology platforms.
Organizations that aspire to divest from the silos of specialization and focus on reducing the incumbent friction and inertia should look past technology and focus on outcomes and ownership. Practically, this can be achieved by organizing teams and specialties by business units and outcomes to avoid traditional technology silos. Through this strategy, teams are not only permitted but encouraged to cross the all-too-often uncrossable silos to ideate multiple solutions before converging on the best one.
Technology executives also need a new operating model and culture that puts the focus primarily on outcomes and empowers the generalist to own the outcome. It is critical not to see this as a technology investment but rather a rewiring of existing investments to maximize returns by creating process efficiencies that emanate from reducing handoffs and specialization silos.
It's important to focus on the philosophical change in your organization’s approach to problem-solving. Analyzing workflows and then reducing or eliminating the low-value functions performed by high-value resources is the first step. These changes, by design, will lead to new ways of tapping into and expanding the value of both the siloed data and your incumbent technology investments.
When appropriately architected, data and technology that is rewired around organizational outcomes can mechanize the operating model and enable iteration, learning and cognitive capabilities. These capabilities will allow you to implement a cognitive learning platform and will allow generalists to move fluidly between silos of specialization, data pipelines and measurements. This structure is amenable to generalists who seek insight into the business not readily apparent to the specialist.
The key to a learning organization is iteration, and a cognitive platform is architected to reduce the "tax on iteration." To facilitate learning, we recommend the cognitive platforms of tomorrow focus on closing the knowledge gaps and practice loops between silos. Removing friction and inertia requires a radical rethinking of current data, technology and development methodologies that have created specialization silos. We believe cognitive platforms should allow data to coalesce around specific outcomes so that generalists can build models, applications and solutions.
Organizations that have spent the last decade warehousing data will be required to utilize and exploit their data using this new and distinctive cross-functional, collaborative model. We recommend strategically disabling the traditional silos in specialization, both from a technology and from a talent perspective. This new operating model, philosophy and technology architecture will do more than automate and optimize workflows; they will become responsive and intelligent. Moreover, instead of wasting cycles in operational handoffs between specialists, the generalist can focus on removing ineffective and inefficient processes imposed on workers by eliminating the low-value workflows from high-value assets.
As an operating principle, when a cognitive platform is paired with generalists who have the full-stack ownership, the output is a high-performing organization.
Comments